Manage Generative AI Risk Before It Manages You
Organizations need to formulate an enterprise-wide strategy for generative AI trust, risk, and security management before deploying applications that use hosted large language models.
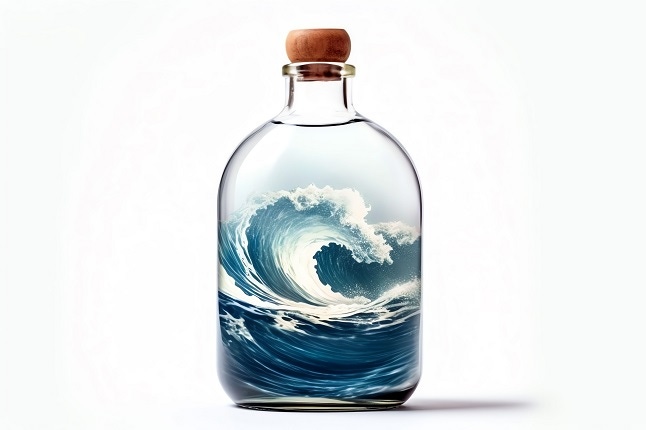
As generative AI innovation proceeds at a breakneck pace, concerns about AI trust, security and risk are rapidly emerging. Hundreds of notable tech and business leaders recently signed an open letter calling for a six-month pause on the training of AI systems more powerful than GPT-4, to assess the safety of these systems. Shortly thereafter, Italy became the first Western country to temporarily ban ChatGPT, citing security and privacy concerns, while in the US President Biden met with the Council of Advisors on Science and Technology to discuss AI opportunities and risks. Most recently, European Union lawmakers called for new rules and regulations for AI tools, beyond those already identified under the region’s proposed AI Act.
The recent open letter is intended in part to give developers time to build in the controls that are needed for users to safely use generative AI large language models. However, the reality is that generative AI development is not stopping. OpenAI could release GPT-4.5 in late 2023, which will eventually be followed by GPT-5 -- which is expected to achieve artificial general intelligence (AGI). Once AGI arrives, it will likely be too late to institute safety controls that effectively guard human use of these systems.
Organizations need to act now to formulate an enterprise-wide strategy for generative AI trust, risk and security management before deploying applications that use hosted large language models (LLMs). Legacy security controls are not sufficient for new generative AI capabilities. Enterprises should continue to experiment, but delay implementation of applications that transmit data to hosted LLMs if they do not have verifiable controls around AI data protection, privacy and LLM content filtering.
The State of the AI TRiSM Tools Market
AI trust, risk and security management, or AI TRiSM, is a framework that ensures AI model governance, trustworthiness, fairness, reliability, robustness, efficacy and data protection.
Broadly, the AI TRiSM tools market is comprised of solutions that fall under four key pillars:
ModelOps, for managing end-to-end model lifecycle governance
Adversarial resistance, for training models to resist malicious attacks
Data/content anomaly detection, for filtering unwanted content
Data privacy assurances, for ensuring privacy for end-users and complying with data privacy regulations
Together, solutions from across these four categories help organizations manage AI model trust, risk, and security. No single platform or vendor currently covers all segments and aspects of the AI TRiSM market.
When applying this framework to applications that rely on hosted LLMs, such as ChatGPT, the full functionality of ModelOps and adversarial resistance can only be implemented by the companies hosting AI models. However, the other two pillars of AI TRiSM -- content filtering and data privacy -- must be managed by users of hosted models and applications.
There are currently no off-the-shelf tools that give users systematic privacy assurances or effective content filtering of engagements with generative AI models. For now, users must rely on LLM application licensing agreements with the hosting vendors to govern the terms of application data confidentiality breaches. Inherited enterprise security controls for doing so are insufficient as they do not apply directly to user interactions with the LLMs. There is a pressing need for a new class of AI TRiSM tools to manage data and process flows between users and companies who host generative AI foundation models.
LLMs require a new class of AI trust, risk, and security management tools
In a recent Gartner poll, 70% of executives said that their organization is in investigation and exploration mode with generative AI, while 19% were already in pilot or production mode. Before taking steps to operationalize hosted generative AI applications, enterprises need to understand LLM risks and the controls required to manage them.
First, enterprises need the ability to automatically filter LLM outputs for misinformation, hallucinations, factual errors, bias, copyright violations, and other illegitimate or unwanted information. Vendors that are hosting these models perform some content filtering for users, but users must implement their own policies and filters to screen out unwanted outputs. This can be done either by building content filtering abilities internally or by engaging with a third party that can provide this functionality.
Organizations also need verifiable data governance and assurances that confidential enterprise information transmitted to the LLM is not compromised or retained in the LLM environment. LLMs are stateless, but confidential information is retained in its prompt history and potentially other logging systems in the model’s environment. This presents vulnerabilities that can be exploited by bad actors or simply by benign configuration mistakes made by LLM system administrators. For now, users must rely on vendor licensing agreements that govern the terms of data privacy breaches.
Finally, users need LLM transparency to conduct impact assessments required to comply with regulations such as the EU’s GDPR or forthcoming AI Act. Organizations under the scope of such regulations have fundamental concerns with LLMs that must be addressed before adopting platforms using these models.
These concerns include:
Privacy Impact Assessments (PIAs): LLMs are a black box, so organizations are challenged to conduct requisite privacy impact assessments without ceding substantial risk to the hosting vendor.
Data residency and data sovereignty: Organizations must understand where LLMs are processing the data they collect, to abide by data residency and sovereignty requirements and preferences.
Legal: If any personal data was used to train the base LLM, then the LLM vendor would have to vouch that they have legal standing in their claims to have eliminated that data from the model’s learnings. Further, under forthcoming rules that are part of the EU AI Act, the LLM vendor will have to disclose copyright materials used to build their system. While such concerns are currently EU-specific, emerging laws and regulations in many other parts of the world will complicate and potentially stall LLM application adoption for similar reasons.
Organizations need to act now to formulate an enterprise-wide strategy for AI TRiSM, particularly as it relates to hosted generative AI applications. The reality is that generative AI development is not stopping, and AI TRiSM has never been more urgent an imperative. It’s time to get on top of AI risks before they get on top of you.
About the Author:
Avivah Litan is a Distinguished VP Analyst at Gartner, Inc. covering all aspects of blockchain innovation and AI trust, risk and security management. Gartner analysts are providing additional insights on AI trust, risk and security at the Gartner Security and Risk Management Summit taking place June 5-7 in National Harbor, Maryland.
About the Author(s)
You May Also Like
How to Amplify DevOps with DevSecOps
May 22, 2024Generative AI: Use Cases and Risks in 2024
May 29, 2024Smart Service Management
June 4, 2024