Selecting a Tech Stack for Your New AI
What to look for when reevaluating your team’s tech stack will depend on where your artificial intelligence solution best fits the team’s workflow. Here are a few ideas.
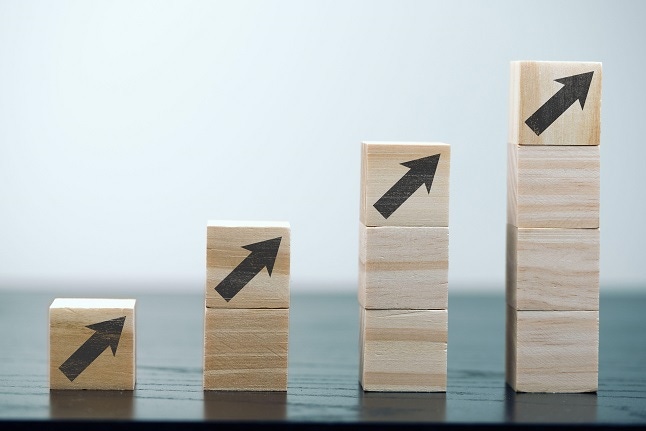
Data has been the new oil over the last several years. Artificial intelligence is now the latest gusher of interest. Thus, a company’s “oil rig” -- its tech stack -- must adjust to gain the new opportunities.
But what should managers select to adjust their tech stack? Should a stack be completely replaced?
The choices are quickly becoming vast. As conference season unfolds, tech giants announce their latest solutions that include AI-based features. Many of these are designed to strengthen the role of data usage within the ecosystems that tech stacks manage. Data is an engineered product, and strategies must be engineered to extract the right value from the data.
But even having a large budget ready for a tech purchase does not bless managers with figuring out the tech stack that best delivers value. Managers sometimes invest in software at the last minute to maintain budget justifications. This leads to buying cloud or desktop solutions that offer limited scope. The result is a stack that implements data in a fragmented manner, a non-unified data management that makes a single source of truth from data expensive to develop.
The ultimate goal of a tech stack is to allow ease of collaboration between business teams, to quickly develop analysis, and to ease maintenance of data visualizations. But delivering these outputs now involves common data interchange formats and protocols. Not every solution does well with a given mix of protocols and applications.
The current AI buzz has captured management’s attention. The latest AI tool updates have made APIs available for building software plugins and for programmatic integration in applications. The updates allow companies to leverage the text generation capabilities of AI. Text generation blends phrases, images, and data to create solutions that fit the prompts. Managers are discovering chatbot-like use cases for the latest AI platforms, allowing text generation to answer questions after examining the media and queries users provide. The ability to use text generation is a major next-level shift from the classification methods machine learning that has so far been used in tech stacks.
Now managers are asking how the output from AI can work within the confines of a workflow supported by a tech stack. So far, the big bets are on the adoption of large language models (LLMs) within AI tools. These have the capacity to remove the workflow that has every professional’s plate overflowing with tasks. Anything to bring better focus matters more than ever.
How to Select a Tech Stack for AI
So, what should business and IT leaders look for in managing a tech stack? A tech stack must connect syntax – the programming that brings data together -- with utility for the users through interface that makes information available.
Four questions highlight what a tech stack decision should address.
How well can data flow within the stack?
What are the pain points for stakeholders within a stack?
What is the ease of integration like if a new tech stack is selected or a new solution is being added?
How easy is it for nontechnical teams to use stack features, particularly prompts if AI is used in the interfaces?
How well a stack answers those questions depends on how well data access by application, file, and storage is managed. An application level is what the user sees at the front-end apps and cloud user interfaces that deliver solutions. The file level is the various access to file backups, such as version control. The storage level centers on where data is warehoused until queried at the application level. Efficient data management across these domains is what makes a tech stack valuable.
AI can enhance how a tech stack delivers across those levels in many ways, such as offering a tagging system for data analysts to organize their data models and dashboards so that stakeholders can navigate these data products easily.
Pricing Models for LLMs: What to Consider
One aspect to keep in mind when looking at any stack features is pricing. With so many solutions trying to add AI, they are relying on APIs from the main providers of LLMs. Some LLM platforms charge for calling data through the APIs or for providing an exclusive premium service like a guaranteed uptime. ChatGPT Plus, for example, costs users $20 a month; users receive better uptime and access to plugins that enhance functionality. Even single-solution makers with a small footprint in AI will introduce premium pricing. Evernote, a note-taking app, is introducing an AI-enhanced search feature exclusively for its subscribers, but it also announced an increased subscription rate from $10.99 to $17.99.
Managers need to keep pricing in mind over the course of an introduction to see if they are deriving true value from the features being introduced.
Many of the AI products will appear as a minimal valuable product framework. This means the features deliver a singular purpose or present limitations. Managers shouldn't overthink their choices on AI features -- if the feature description does not strike a manager’s imagination in how the solution can improve administrative workflow, then managers should look further for better options.
Leaders of IT projects face the same priority with AI-enhanced tools as they do with all solutions -- to always seek tools that enhances a holistic workflow among teams and that aligns with organization priorities. Otherwise, a low-stack adoption among workers occurs, leading to a potentially low ROI on a stack investment and a tech stack bloat -- solutions becoming a cost overhead because of low usage.
If managers are seeking to be the “wildcatters” in the AI era, they must be better at asking how the opportunity best serves their organization for the long haul.
What to Read Next:
Spring Cleaning the Tech Stack
Pairing AI with Tech Pros: A Roadmap to Successful Implementation
About the Author(s)
You May Also Like
How to Amplify DevOps with DevSecOps
May 22, 2024Generative AI: Use Cases and Risks in 2024
May 29, 2024Smart Service Management
June 4, 2024